Professor |
||
Dates |
12-16 September 2022 |
|
Hours |
15:30 to 19:00 CEST |
|
Format |
In person |
Intended for
This course is intended for anyone who needs to run and/or analyze experiments under spillovers or other forms of cross-unit interference.
Prerequisites
Graduate training in econometrics, statistics, or a related field is recommended as a prerequisite.
Overview
Randomized controlled trials are typically studied under a “no interference” assumption, whereby the treatment given to one experimental unit does not affect outcomes for others. In recent years, however, there has been considerable interest in running experiments in complex systems where cross-unit interactions not only exist, but are of central importance. Examples of such settings include community-level interventions in development economics, and interventions that affect production/consumption decisions of participants in a marketplaces or online platform. This series of lectures will survey recent advances in experimental design under cross-unit interference, with special emphasis on settings where interference can be captured via economic modeling.
Topics
Review of treatment effect estimation without interference. Randomization, potential outcomes, unconfoundedness and efficiency
Introduction to treatment effect estimation under interference. Cluster-randomized experiments. Average direct and indirect effects. The network interference model
Interference via general equilibrium effects, including price and congestion effects
Treatment effect estimation under strategic behavior, and interference via competition
Introduction to heterogeneous treatment effect estimation and targeting, including via machine learning based methods. Spillovers and externalities in optimal targeting
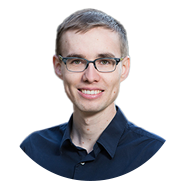
Stefan Wager is an associate professor of Operations, Information, and Technology at the Stanford Graduate School of Business, and an associate professor of Statistics (by courtesy). His research lies at the intersection of causal inference, optimization, and statistical learning. He is particularly interested in developing new solutions to problems in statistics, economics and decision making that leverage recent advances in machine learning.