CEMFI Summer School
New Developments in the Econometrics of Heterogeneous Workers and Firms
Instructors
Dates
1-5 September 2025
Hours
15:00 to 18:30 CEST
Format
In person
Practical Classes
No
Intended for
Researchers, economists, and policy practitioners.
Prerequisites
Participants should be comfortable with a Master’s level course in econometrics.
Overview
In recent years there has been an upsurge of interest in new methods for the analysis of matched employer-employee data to study the labor market, partly motivated by the increasing availability of this type of datasets. Existing methods are also finding applicability in other areas such as international trade, economic geography, environmental economics or intergenerational mobility. A main theme is how to deal with multiple heterogeneities and their potential interactions. This course will introduce newly developed approaches to deal with unobserved heterogeneity in conventional and matched panel data sets with an emphasis on discrete-classification methods. It will also provide an overview of traditional methods to decompose earnings variability into worker and firm-level effects as well as the more recent distributional approaches. Finally, the course will review methods for studying transitions and dynamic responses.
Topics
- Introduction to clustering methods in panel data analysis
- Grouped fixed-effects estimation
- AKM decompositions of two-sided heterogeneity from matched panel data
- Applications to labor markets, international trade, and the environment
- Distributional approaches for linked employer-employee data
- Models of worker and firm dynamics
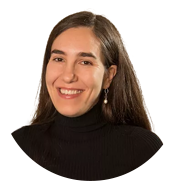
Elena Manresa is a tenured Associate Professor at the Department of Economics of New York University and a Sloan Foundation Research Fellow. She obtained her PhD from CEMFI in 2014. She was on the faculty of MIT Sloan School of Management between 2014 and 2017. She has also been a visiting professor at Stanford GSB, the University of Chicago, and Yale University. She has published her work in leading journals like Econometrica and the Journal of Econometrics.