CEMFI Summer School
Data Science for Economics: Mastering Unstructured Data
Instructors
Dates
18-22 August 2025
Hours
9:30 to 13:00 CEST
Format
In person
Practical Classes
There will be between two and three voluntary sessions in the afternoon (from 15:00 to 17:00) led by a teaching assistant. Exact dates will be announced before the beginning of the course.
Intended for
Academic researchers, policy analysts, data analysts, and consultants who are using, or who wish to use, unstructured data sources such as text, detailed surveys, images, or speech data in their work.
Prerequisites
A basic familiarity with probability and statistics at advanced undergraduate level. The hands-on classes will require students to work through Python notebooks that will be prepared in advance. Extension problems will involve the modification of these notebooks, which requires familiarity with the basics of Python. An introductory session to Python will be provided by a teaching assistant. Therefore, previous programming experience in other languages is sufficient.
Overview
Over the past decade, the use of unstructured data - such as text and images - has grown significantly in economics and related disciplines. The emergence of large language models (LLMs) like ChatGPT, as well as broader advancements in generative AI, has transformed how researchers analyze and interpret these data sources. These technologies not only enable text classification and sentiment analysis but also facilitate more complex tasks such as text generation, forecasting, and model fine-tuning. As a result, researchers now have unprecedented opportunities to extract insights, automate tasks, and develop AI-enhanced economic models.
By combining practical implementation with intuitive theoretical insights, this course prepares participants to effectively leverage unstructured data, large language models, and generative AI in economic research. Participants will gain hands-on experience in fine-tuning LLMs, developing AI-powered analytical pipelines, and using generative models to push the boundaries of modern economic analysis.
The course is structured around five key components:
- Analytical Techniques: Key statistical and machine learning methods for analyzing unstructured data. Topics include Bayesian updating, matrix factorization, and predictive modeling using neural networks and random forests. Special attention will be given to how these techniques apply to natural language processing (NLP) and generative AI. Rather than focusing on technical derivations, the emphasis is on developing an intuitive understanding of these algorithms and their applications.
- Large Language Models (LLMs) and Generative AI: Architecture, training methods, and practical applications in economics. Topics include:
- Text embeddings and transformers: How models like BERT, GPT, and LLaMA process and generate text.
- Fine-tuning and prompt engineering: Customizing LLMs for domain-specific economic research.
- Generative AI for synthetic data: Using AI to create simulated datasets for economic modeling and forecasting.
- Challenges and biases: Addressing interpretability, fairness, and limitations of generative models.
- Economic Applications: topic modeling and sentiment analysis to analyze policy debates and financial markets; Fine-tuning LLMs for economic forecasting and macroeconomic policy analysis; generative AI for survey automation and synthetic data generation.
- Hands-on Implementation: Through guided coding sessions, students will develop the skills to integrate AI-driven techniques into their own research projects. Participants will work with real-world datasets using Python and Hugging Face Transformers. They will learn fine-tuning techniques for LLMs, including training models on domain-specific text (e.g., financial reports, economic policy papers). They will implement custom NLP pipelines to analyze economic data.
- Data Collection and Preparation: Methodologies for collecting, processing, and structuring unstructured data for AI-driven analysis. This includes web scraping and APIs for extracting textual and financial data, preprocessing pipelines for cleaning and structuring data for machine learning models and ethical considerations when working with LLMs and AI-generated data.
Topics
- Topic modeling and probabilistic approaches: Latent Dirichlet Allocation (LDA)
- Large language models: Transformer architectures, pretraining, and fine-tuning for domain-specific tasks
- Evaluating AI predictions: Accuracy, precision-recall, and interpretability in unstructured data models
- Image analysis and classification: Convolutional neural networks (CNNs) and transfer learning
- Web scraping and automated data extraction from online sources
- Speech-to-text processing and sentiment analysis of spoken language
- Generative AI: Text generation, synthetic data, and AI-assisted research methods
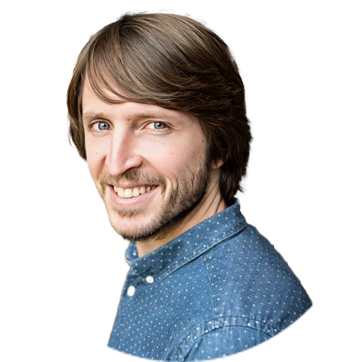
Christopher Rauh is an ATRAE Distinguished Researcher at the IAE (CSIC) and a Professor of Economics and Data Science at the University of Cambridge. He is also a Fellow of Trinity College Cambridge, an Affiliated Professor at the Barcelona School of Economics, an Associate Senior Researcher at PRIO, and a Research Affiliate at CEPR, HCEO, and IZA. His research focuses on designing surveys and analyzing unstructured data using machine learning. He serves as Principal Investigator at conflictforecast.org and EconAI and has led multiple projects in collaboration with the FCDO, the German Foreign Office, and the IMF. Additionally, he is an Associate Editor at the Economic Journal and has published in a wide range of economics and political science journals, as well as in international media outlets.